Big Data, Big Problems? Resources For Applying Big Data To Your Business The Smart Way
I’m the least-qualified LunaMetrician to be writing about the field of Data. I’ve never had a great recall of numbers, I designed much of my time in academia to avoid spreadsheets and focus on the arts and I even dropped an early college Statistics class. However, I’ve always been fascinated by grand narratives, Economics and business trends. Big Data’s story is still being written, and what has been committed so far is fascinating. I love reading trends and case studies to see how Big Data is impacting the way we do business as SEO and Analytics providers and how it can benefit my roster of clients. Let’s look at ways your company can approach and benefit from these techniques.
Several years ago, Big Data’s pitch to the business world went something like this:
- Collect Everything
- Apply Computational Processing Power
- ????
- Profit
This plan of action has since been lampooned in a Dilbert strip, surely the official coronation ceremony for any major business trend.
There was a bit of an assumption that if you just got more data, you could magically intuit patterns that would allow you to crush the competition. To some extent this has come true with highly complex statistical models & algorithms. Amazon can easily discern your shopping habits and serve you related products. Netflix knows that you marathon every Gossip Girl season and makes contextual recommendations even though you swear to your friends you’ve never seen it. It’s rumored that Facebook knows when you’re dating someone new long before you change your relationship status, based on the frequencies of your interactions with a person new to your network. As internet users, we create mounds of data for the big boys to process. But your business doesn’t have a server farm the size of Amazon’s. You probably don’t you have a team of PhD sandbox economists on staff to build models and discern your data, but you can still benefit from Big Data’s powers and promises.
Let’s look at what you need to know:
The Benefits
There are some great advantages in incorporating Big Data and advanced analytics into your business decision making. A 2013 Bain survey of executives from 400 large worldwide companies yielded the following insights. Companies incorporating advanced analytics and Big Data techniques reported advantages in financial performance and advantages in decision making.
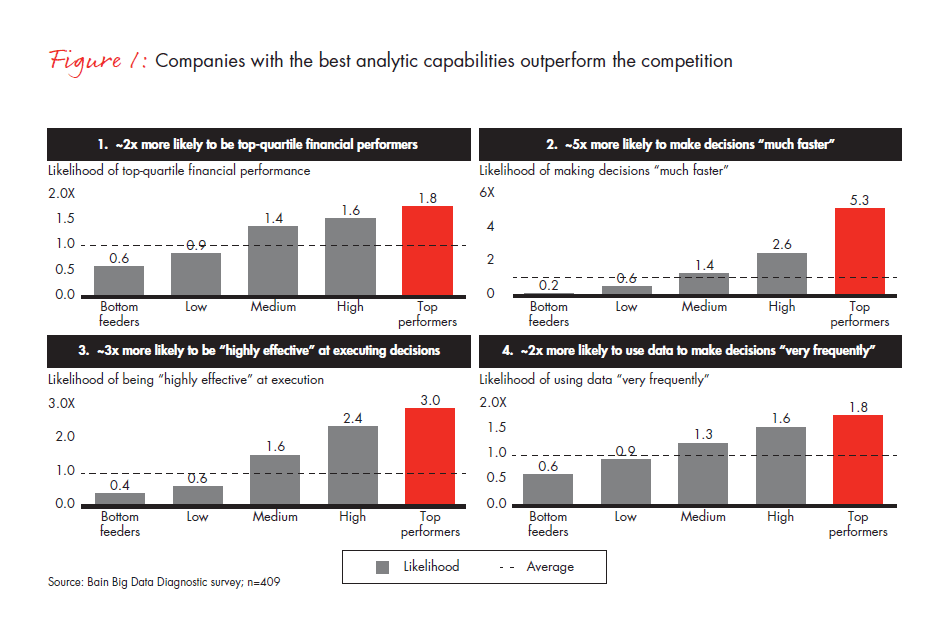
The Buzzword Problem
To address concerns about defining “Big Data”, I consulted LunaMetrics’ own Data Evangelist Jonathan Weber. His take:
First, I think “big data” is an over-used and over-hyped buzzword. What it really means is a set of tools and processes for dealing with truly huge datasets — datasets that are so large they can’t be dealt with well by traditional database systems and applications. Many companies don’t really have this problem, but what they hear when someone says “big data” is simply: We have a bunch of data stuck in various places and we don’t know what to do with it all. Which is a highly interesting and relevant problem, but it doesn’t necessarily fit the definition of “big data”. I like the term “data science”, meaning ways of extracting knowledge from data, which can encompass all of those types of data problems, from big to little.
This is of course a great point. To apply a true big data solution, it helps if you are dealing with really big data. You can however apply similar techniques to any size dataset.
Sampling Bias
In FT.com’s Big Data: Are We Making a Big Mistake, Tim Harford looks at some common failures of Big Data applications that we don’t often hear about, like sampling bias and false positives. Harford discusses the issues in sampling that Big Data creates and provides some terrific examples, discussing Google Flu‘s successes and failure as well as Target’s ability to determine if a customer is pregnant based on purchase habits (Famously profiled in 2012 by the New York Times – How Companies Learn Our Secrets).
Big Data represents a massive shift in statistical sampling simply by having all the data. Harford explores sampling bias in a great anecdote from the 1936 US Presidential Election:
“In 1936, the Republican Alfred Landon stood for election against President Franklin Delano Roosevelt. The respected magazine, The Literary Digest, shouldered the responsibility of forecasting the result. It conducted a postal opinion poll of astonishing ambition, with the aim of reaching 10 million people, a quarter of the electorate. The deluge of mailed-in replies can hardly be imagined but the Digest seemed to be relishing the scale of the task. In late August it reported, “Next week, the first answers from these ten million will begin the incoming tide of marked ballots, to be triple-checked, verified, five-times cross-classified and totalled.”
After tabulating an astonishing 2.4 million returns as they flowed in over two months, The Literary Digest announced its conclusions: Landon would win by a convincing 55 per cent to 41 per cent, with a few voters favoring a third candidate.
The election delivered a very different result: Roosevelt crushed Landon by 61 per cent to 37 per cent. To add to The Literary Digest’s agony, a far smaller survey conducted by the opinion poll pioneer George Gallup came much closer to the final vote, forecasting a comfortable victory for Roosevelt. Mr Gallup understood something that The Literary Digest did not. When it comes to data, size isn’t everything.
…But if 3,000 interviews were good, why weren’t 2.4 million far better? The answer is that sampling error has a far more dangerous friend: sampling bias. Sampling error is when a randomly chosen sample doesn’t reflect the underlying population purely by chance; sampling bias is when the sample isn’t randomly chosen at all. George Gallup took pains to find an unbiased sample because he knew that was far more important than finding a big one.”
In SEO, this can happen quickly. Companies can measure the wrong things or not filter their website data properly and falsely conclude traffic is moving one way when it may really be moving another. I encourage you to read the full piece! It’s a terrific primer on the issues facing companies integrating big data.
Correlation vs Causation
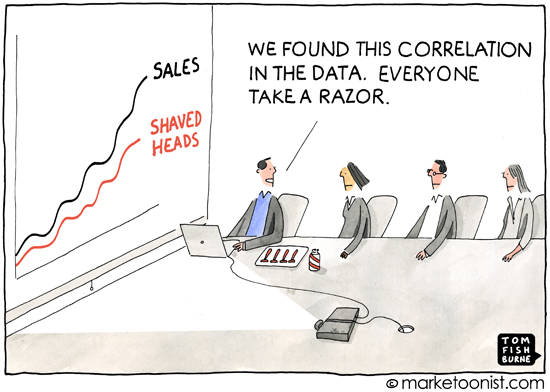
This cute Tom Fishburne cartoon accurately summarizes the problems you get when you confuse correlation with causation. Correlation simply suggests a relationship between two things. In the chart in the comic above, it’s clear that Sales increased over the same time period as the popularity of Shaved Heads rose. The presenter incorrectly defines the cause of one to be the other. If shaved head popularity would increase sales does that mean the inverse relationship would also occur? Imagine the presenter saying “If the company goes bankrupt and sales slow to a trickle, we are confident people will grow hair again”. Wait…what? It’s a very silly assumption. So is the original one. Or, as my co-worker Andrew Garberson said about the comic, “Hey, we’re doing great for (not provided), better focus on that keyword!” In the video below the Freakonomics guys (Stevhen Levitt and Stephen Dunbar) expertly explain Causation & Correlation in terms everyone can understand in a short 3 minutes.
I Can Just Automate Everything and Let Algorithms Run My Business. Right? Right?
Who doesn’t want to replicate the success of Amazon, Facebook, Netflix or Wall Street? Once you’ve attained a large enough data set and observed relationships, the next natural step is to automate the process, causing a series of events to trigger automatically. MIT professor Kevin Slavin presented an excellent overview of the Algorithmic World we now live in at TED. It’s clear that hiccups can occur and automated actions can leap before we can stop them. Kevin’s Amazon example of the wildly out-of-control used magazine price is a terrific example. This is something that is easy to catch and correct when a person is updating your website, but harder when the changes are arithmetically-driven.
Even the almighty Google relies on human eyes and hands to comb websites, and rate their experience to determine what common factors should enhance the rankings of websites before scaling that knowledge with machine learning/Big Data techniques. Google finds that context is important in determining things, and humans are pretty great at that. There’s just no replacing the human brain (yet). Your business can benefit from an expert’s eye on evaluation of your data, marketing techniques and KPIs.
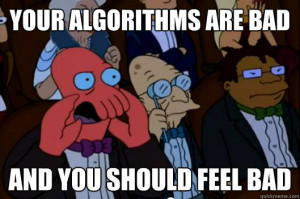
Poor Data Quality
It’s happened to me over and over again. A new SEO client approached me excited about rising organic traffic, then after some investigation, I have to let them know that traffic is actually mis-attributed paid traffic. Poor data attribution is bad. Poor data attribution in a huge data set is worse. To really get the most out of Big Data-style crunching, your input data has to be great. I learned an old axiom in Film & Video Production class that has also served me well working with data: “Garbage in, garbage out.”
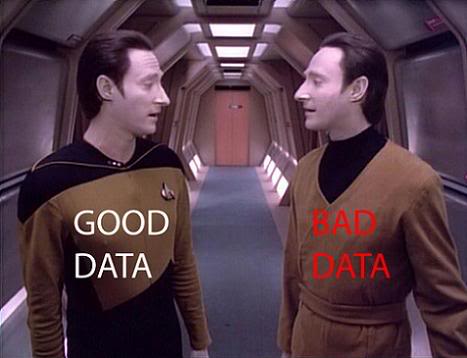
Jon Meck of the LunaMetrics’ Analytics team said the following:
A major obstacle with any sort of data collection, and especially “big data”, is how to pull actionable conclusions out of the noise. What are the questions you are hoping to answer, and will the data you have collected help you with that?
Optimizing for the Wrong Goal
With so much data flooding in, what do you focus on? Lasering in on the top one or two most-profitable products or most popular services may cost you in the long run. It’s a very natural conclusion to jump at the best-performing item and reconfigure your whole business to attack only that, or shave your head to induce more sales like in the comic above. This could be short sighted, due to what’s known as the Long Tail. Popularized by Wired writer Chris Anderson, the Long Tail concept suggests that the most popular or best selling items may not represent the most aggregate volume. This has been applied from everything to the music industry to candy sales, but as you see in the chart below from Moz, it certainly applies to SEO traffic & Keyword Value.
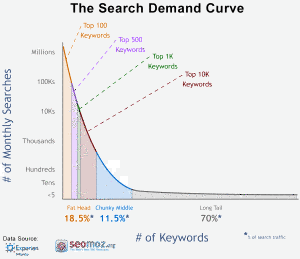
In the chart above, the website receives 70% of its monthly search traffic from long tail keywords, not the most popular keywords. Sure, you should continue to focus on top terms, but not at the peril of ignoring the majority source of your traffic. This makes establishing goals and expectations from your big data queries even more important. We all want data to make us feel more secure about business decisions, but are they pointing the right way? This should be carefully considered before implementing a plan. What are your bottlenecks? Where will you reap the most value from new-found data insights? Answering these questions will maximize your gains.
As the online world continues to push towards automation and optimization, there is still value in looking at the big picture, and that requires an awful lot of critical thinking, something Big Data is not yet doing well.
Next Steps?
If you’re interested in Big Data approaches to Google Analytics data, contact us about BigQuery for Google Analytics and LunaMetrics’ experts will be happy to answer your questions and explain how these techniques can provide actionable insights for your business. Below I’ve collected some great resources if you’d like to learn more about Big Data and its effects on the business landscape.
Reading List:
Opinion / Reports
Wired.com – Forget Big Data, Think Long Data – by Samuel Arbesman
Guardian – Why Lean Data Beats Big Data – by Matti Kaltenan
Scientific America – Saving Big Data from Big Mouths – by Cesar A. Hidalgo
Harvard Business Review – Google Flu Trends’ Failure Shows Good Data > Big Data – by Kaiser Fung
Bain – The Value of Big Data PDF – by Rasmus Wegener and Velu Sinha
McKinsey – Applying Advanced Analytics in Consumer Companies – by Peter Breuer
Deloitte – Big Data: The Three Minute Guide PDF by Forest Danson
Fox News – Beyond Big Data: Why Human Interpretation Still Counts by Nicole Fallon
Instruction
LunaMetrics – Google Analytics & BigQuery: The Why’s & How’s – by Jonathan Weber
LunaMetrics – Google Analytics Premium & BigQuery: Access Raw Data in Seconds – by Dorcas Alexander
CMO.com – Five Ways to Map Big Data to Business Goals – by Joe Cordo
Recent News
CNNMoney – Big Data Knows You’re Broke – by Melanie Hicken
Forbes.com – Prolonging Machine Life and Efficiency with Big Data – by Russ Banham
Services
Ready to implement Big Data for your business? Qualified Data Consultants can help you evaluate accessible platforms. Check out these services: