Common Reporting Pitfalls In Google Analytics
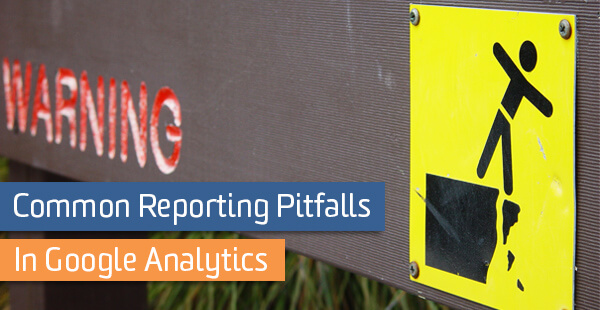
You’ve implemented Google Analytics across your website and have lots of great data coming in. Now what? It’s important to be able to turn that data into useful insights for your organization. This involves key skills like knowing how to ask the right questions and understanding what your data means. In this post, I’ll describe a few common pitfalls in the world of Google Analytics reporting – and how to avoid them!
Sessions by Page
Suppose you have several important pages on your site, and you want to know the number of session in which each page received views? This is a common situation that often leads to reporting mistakes. To answer this situation, many people use a combination of the dimension page with the metric sessions.
However, the metric sessions means something different in this context. In fact, the metric sessions is roughly equal to the metric entrances. That is, a page receives a session if it is the first page the user viewed during that session. Pages further along in the user flow will have zero sessions.
Instead, try using the metric unique pageviews. Unique pageviews measures the number of many sessions during which that page was viewed. After a given session, a page has zero unique pageviews if it was not viewed by the user, and one unique pageview if it was. Viewing the page multiple times will not increase the unique pageviews count over one. This is exactly the metric we are looking for.
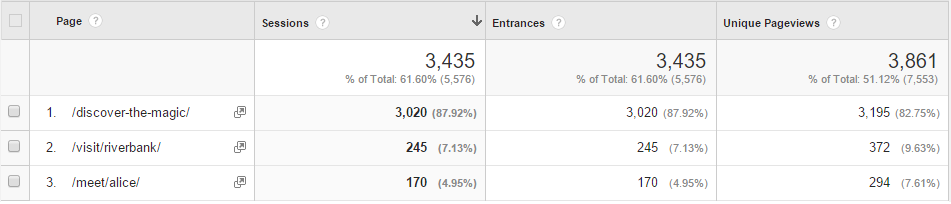
Users by Source or Medium
Another common reporting problem is analyzing your users by traffic channel. For example, you may want to know what percentage of your users come from organic search. On the surface, this seems like an easy question. However, let’s dig a little deeper. What does it mean for a user to be an organic user versus a paid search user?
After all, a single user could come to your site through a variety of different traffic channels over time. If a user first finds your site through a paid advertisement, and later comes back through organic search, how would you classify that user?
One way to analyze this question is to look at the dimension source / medium combined with the metric users. However, this can lead to some surprising results if you do not think through what you are looking at. Let’s take a look:
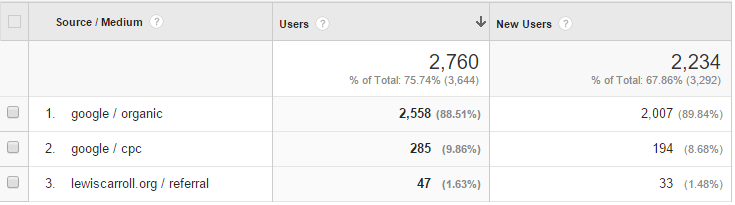
Try summing up the users across all traffic sources. You will get a total of 2,558 + 285 + 47 = 2,890 users. This does not match 2,760, the total number of users Google reports (at the top of the chart). Why is this?
In the Google / organic row, you can see the total number of users who have used a Google organic search to find our site. In the row for Google / cpc, you can see the total number of users who used AdWords to view our site.
However, if the same user comes to our site multiple times, once through organic search and once through paid advertising, that single user will be counted in both rows. This will make aggregating your user numbers tricky if you export these numbers into Excel. It may also be a sign that you are looking at the wrong metric.
It’s time to rethink your real question here. Are you looking for channels that drive the most traffic to your site? If so, you should use the sessions metric. Perhaps you care about how users originally discovered your brand. In which case, use the new users metric. Maybe you actually care about which channels are driving conversions on your site. In this case, check out the multi-channel funnel and attribution reports.
It’s fine to look at a report that shows users by traffic channel, but make sure you understand what you are looking at!
Averages of Averages
One common reporting use case is to pull a set of data that includes several dimensions. For example, you might download a report detailing the bounce rate to your site by landing page, country, and traffic channel. Then you might use this data to create a dashboard using your favorite visualization tool, like Excel or Tableau. During this process, you would need to aggregate your data in multiple different ways. This opens up the potential for computational errors.
One common error is aggregating rates and averages. I will discuss bounce rate in this post but the same methodology applies to other aggregated metrics like exit rate, pages per session, and average time on page.
What happens when you break out the bounce rate by landing page?
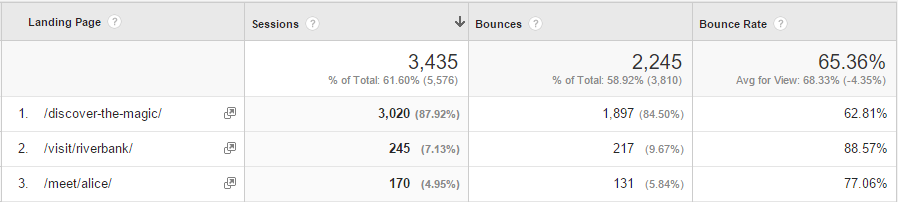
Notice that Google displays the overall bounce rate as 65%. However, if you average the bounce rates of each landing page, you end up with (62.81% + 88.57% + 77.06%) / 3 = 76.15%. Why are these numbers different?
The 65% bounce rate reported by Google represents how your site performed as a whole. In total, your site received 3,435 sessions, of which 2,245 of those were bounces. As a result, your site-wide bounce rate is 2,245 / 3,435 = 65%.
The 76% bounce rate that you computed represents how a “typical” landing page on your site performs. Some of your landing pages have lower bounce rates, around 62%, while some are less effective and have higher bounce rates of 88%.
However, on average, your landing pages have a 76% bounce rate. Note that in this computation, pages with high visibility are weighted equally with pages that received smaller amounts of traffic. If you get fancy and weight your pages by the number of sessions they received, you will end up computing the overall bounce rate (65%). Try it!
Which number should you use? When in doubt, go with the overall site bounce rate (65%). Most dashboards are designed to show big picture numbers of overall site performance. If you display the “landing page” version of bounce rate out of context, your report will be confusing and misleading.
In order to compute this number correctly, you will need to think ahead when downloading your data. Remember, simply averaging the bounce rates will give you the wrong overall number. So if you only download the bounce rates along with the landing pages, you will never be able to compute the correct bounce rate.
Instead, you should download the number of sessions and bounces associated with each landing page. Then add up the total number of bounces and sessions. Finally, divide the total bounces by the total sessions. The bounce rate for each landing page can also be computed by dividing the bounces on that page by that page’s sessions.
For example, in Tableau you can create a calculated field for bounce rate:
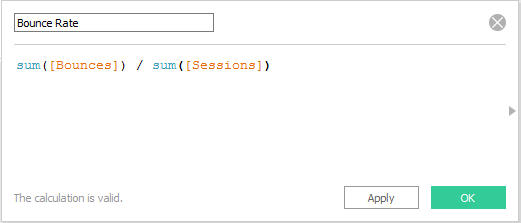
But what if your report focuses on landing page performance?
In this case, using the average of bounce rates may be a good option for you. However, I would caution you to consider a few things:
- What set of landing pages should I include in my analysis? Different types of pages on your site naturally have different performance . You should consider grouping pages with similar goals together.
- Did all of these pages get a significant amount of traffic? If certain pages only received a few sessions, you should be careful about including them in the analysis. For example, a landing page with only 1 session will either have a bounce rate of 100% or 0%. This is not representative of the nature of the page, but simply a fluke due to small traffic numbers. I would recommend excluding any pages that have too few sessions for your site. Pick a number that works for you, 30 sessions, 3000 sessions, it will depend on your site and your traffic. If this is not possible, make sure you think through what your numbers mean.
- Are any outliers overly impacting your results? Many outliers will be excluded by restricting your analysis to pages with significant amounts of traffic. However, if your numbers are still skewed by extreme outliers, you should consider using a more robust statistic, such as the median.
Parting Advice
As always, think through your questions carefully, and make sure you know what those numbers mean! With good data and careful thought, you can bring great insight to your organization.