Checklist For Analyzing Website Test Results
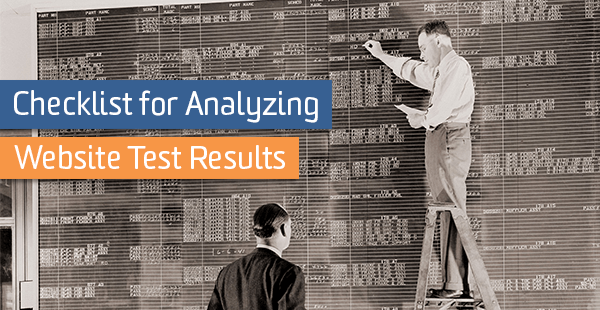
If you are like me, you are bombarded by ad-hoc analysis requests. How are the new landing pages doing? Are the paid ad campaigns successful? Which template performs best? Which of these content types is more popular? Was our site redesign worth the millions we paid for it?
These requests sometimes involve high stakes decisions and may lie outside the bounds of your standard reports and dashboards. While some of these answers are easy – Is anyone using our mobile site? – others require extra time and care. Before you send off your final analysis, use the checklist below to avoid some common analysis mistakes.
Do You Have One Success Metric?
This is the most critical, and often most difficult, step in the analysis process. Buzz words like engagement, brand-awareness, or conversion sound great in emails, but unfortunately are useless in the world of testing. To design a meaningful test, you must identify one specific action that you are optimizing for.
Here are some examples: completed transaction, file download, button click, view of a certain page, or total revenue collected. If you are unsure of which metric to use, go back and ask what the primary goal for this initiative is.

What are the Trials in Your Test?
It is important to know what the “test subjects” are in your analysis. In statistics, we sometimes refer to these “subjects” as trials. Each trial should represent a single opportunity for success. What you choose as a trial might vary based on your objectives and your company.
Hint – if you are optimizing for a conversion rate, bounce rate, or some other percentage, this will be the denominator of your rate. Here are some examples:
- Sessions – This is default for Google Analytics goals, which measures the number of “converted sessions”.
- Entrances – This is a great metric to use if you are doing landing page optimization. Note, this metric is very similar to sessions, but will be a little more intuitive for analysis of landing pages.
- Users – If you have a lengthy buying cycle with multiple user interactions before a conversion, you should consider defining each trial as an individual user.
- Pageviews – If the user can convert on that page – and you hope they will convert every time they view the page – use pageviews as your trials. Also a good metric to use if you are measuring exit rate.
- Pages – In certain cases, your “test subjects” might be pages on your site. If you have questions about comparing the performance of content types or topics, you should aggregate your analysis around each page, rather than a user- or session-based analysis.

Is There Enough Traffic to Run a Test?
In order for your results to be statistically significant, you will need significant numbers of both trials and successes. This means your site needs to be getting a fair amount of traffic and your conversion rates can’t be too low. The exact amount of traffic you need depends on a number of factors, including the statistical test you are running, the difference in performance of your pages, and the power and significance that you wish to achieve with your test.
As a general rule of thumb, I recommend having at least 1,000 trials for each variation and enough successes that adding or subtracting a few conversions will not have much of an effect on your results. The more traffic you have, the easier it will be to distinguish between similarly performing variations.
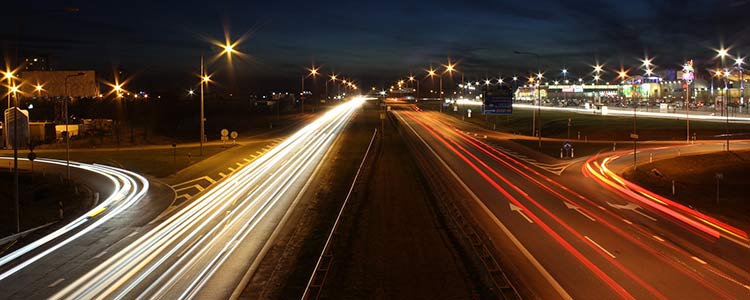
Are There Confounding Factors?
Confounding factors are probably the most dangerous issue you can encounter when running a test. So what is this snake in the grass? A confounding factor is an outside influence that could skew the results of your test. Can you reasonably expect each of your trials to have the same chance of succeeding? Or, are there other factors at play that could affect your results? Here are some examples:
- Traffic Sources – Traffic to landing page A is from social sources while traffic to landing page B is from paid ads. If landing pages A and B have different conversion rates, is it due to the different traffic sources or the different content on the page?
- Seasonality (Holidays) – Your site redesign went live on January 1. The conversion rates in January on the new site are much lower than the conversion rates in December on the old site. Is this difference due to the site redesign, or to the increase in purchases over the holiday season?
View this great post for more examples and suggestions for dealing with these pesky confounders.
Is Each Trial Independent?
This issue is a little more subtle. Most statistical methods require that the trials are independent, which has a very specific, technical meaning in the world of statistics. For our purposes, you need to answer the question: “Are any of my trials affecting each other?” In order to answer this question, you need to make sure you have a good understanding of what your trials are.
Here are a few examples where independence fails:
You are measuring conversions per session (each session is a different trial). However, you have a lot of return visitors. Whether a visitor converted in the past may be affecting whether they will convert in the future. As a result, future trials (sessions) of a user are affected by past trials (sessions) of the same user.
You are measuring which types of users are more likely to comment on your articles. (Each user is a different trial.) However, users might be more likely to comment on an article if other users have already done so. As a result, a user’s commenting behavior will affect the behavior of other users that view the same articles.
Are There Unintended Consequences?
Although we are testing for the success or failure of a single metric, it is important not to get tunnel vision. Test winners will spell disaster if they break your e-commerce check-out process. Make a cheat sheet of your site’s most important metrics; then doublecheck that the test is not hurting your business’s bottom line. Even if you decide that the good outweighs the bad, be sure to include possible negative consequences in your analysis.
Did You Save Your Analysis?
Make sure to record the steps you used in performing your analysis. At a minimum, you should keep track of the following parameters:
- Date range of test
- Details around your data sources (list of dimensions and metrics used, along with any filters or segments – reference to a query or specific report is great)
- Be sure to detail the source of any data external to Google Analytics as well. (What is this data and how did you get it?)
- Raw data files
- List of any steps used to process the data
- Statistical test used (and results)
You may not include all of this information in your presentation of the test results. However, when you start getting questions around the details of your analysis, you will be glad you took these notes. This can also be helpful if you are asked to “refresh” your analysis with updated data.
Explain Your Results and Be Confident
Make sure you explain your results in a way that is meaningful to your audience, and be confident in your story!