Why Should I Run A/B Tests On My Website?
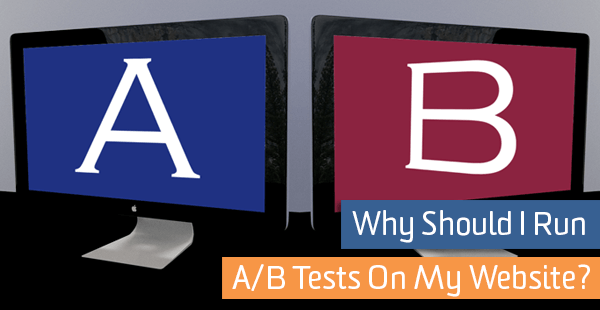
Today’s world is all about optimization. As a digital marketer, you may be tasked with optimizing content, ad targeting, email frequency, and so much more. Although you value making data-driven decisions for optimization, you probably already have access to a mountain of data about your website.
If you already have reliable Google Analytics data, is purchasing another “data-driven” tool really worth it? Can Optimizely or the new Google Optimize 360 give you more valuable data than you already have, and are the test results really valid?
Why Experiment When You Already Have Some Data?
I want to take a step back and draw an analogy to the medical field. Randomized trials are well established in that domain.
Medical researchers get data by conducting observational studies or analyzing existing data sets. This gives researchers information about how medicine is practiced in the real world without the intervention of a randomized study design. However, within this observational data, there can be significant influence from confounding factors.
For example, people who don’t smoke, exercise frequently, and eat healthy may also be more likely to use a specific drug. People who smoke, are couch potatoes, and eat fast food may be less likely to take this drug. When you look at the data, it is difficult to tell if any differences in health outcomes were due to smoking, exercising, eating, or the effectiveness of the drug.
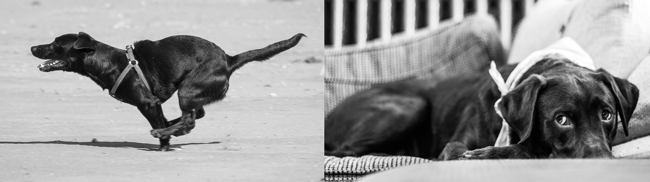
Eliminating Confounding Factors with Random Selection
The medical community addresses this statistical concern by conducting experiments such as randomized controlled trials. This process randomly selects people into two different groups – treatment and control. The idea is to split up the smokers, the couch potatoes, and the fast food lovers into different groups. The randomization should also help slit up all of the other demographic and lifestyle factors that the researcher may not even know to check for.
Factors that could have caused bias in our observational study are now just random noise within the experiment. The whole idea of randomization is to turn systematic error, which is hard to account for, into random error, which is much easier to deal with statistically.
Because of the randomization process within clinical trials, researches are not concerned that all of the people in the experiment are exactly the same. Medical researchers don’t clone one person and stick them into a laboratory in order to make sure that all trials are the same. That might lower the variance within the results but it would also mean that the drug testing is hyper-targeted towards one person. The results might not generalize well to the rest of the population.
Instead they accept that different people have different genetics, environmental factors, etc. If the randomization is done correctly, they can assume that these factors will show up by increasing the variance, but not as biasing one or other of the drug options.
How This Applies to your Google Analytics Data
The data that we have in Google Analytics is similar to data from an observational study. Within Google Analytics, we measure how people interact with our site without the influence of an experiment. This data is valuable and can give us a starting point for deciding where there is room for optimization. However, just like in the medical community, we are faced with the issue of confounding factors.
For example, in our Google Analytics data, users on desktops may be likely to view page A and also more likely to convert. Users on mobile devices may be more likely to view page B and also less likely to convert. We notice that conversion rate on page A is higher than that of page B. Is this difference in conversion rate due to the quality of the page, or the difference in device used?
Whenever we analyze our Google Analytics “observational” data, we have to worry about many different confounding factors: desktop vs mobile, different traffic sources, geographic effects, etc. But when we run an experiment, those differences are taken care of for us. We no longer have to worry if differences in performance are due to some underlying factor that we did not account for.
We don’t have to hyper-target our audience or use fancy statistical techniques to reduce the effects of confounding. Instead, we can target our experiment to the audience it was designed for, and let the randomization take care of the rest.
Where Can A/B Testing Go Wrong?
However, even when we randomize our experiment, there can be some systematic error. In clinical trials, one example of systematic error is when the patient knows whether or not they are getting the drug. This knowledge could affect patient health and bias one group in a different way than the other. Medical researchers must deal with this type of error directly within the experimental design. One common solution is to use sugar pills or placebos for patients not receiving the treatment.
Online experiments can also experience systematic error. Listed below are a few examples:
- Flicker – Your pages may experience a flicker or delay on the page due to improperly implemented experiments. This can bias one variant over the other in an artificial way that will skew your test results.
- Coding Issues – If you do not properly QA both of your variants, you may find that one variant does not display properly on certain devices or browsers. This could negatively affect only one of your variants and skew your test results.
- Changes Over Time – If you use testing software that changes the percentage of traffic sent to each variant over time, you should ensure that this does not bias one variant unfairly. For example, you should make sure that both variants receive weekend and weekday traffic. Also, any holiday, sale, or deadline based traffic should be equally distributed between the two variants. Also, make sure that the time period during which you run your experiment has similar characteristics as the time during which you will publish your changes.
- Change Aversion – Loyal users may be confused by a new layout, navigation, or branding on your site. It might take them a while to get used to the new changes, even if these changes end up being for the better. This would give an unfair advantage to the control/old variation.
- Novelty Effect – Users may be excited about a new and shiny feature on your site even if it does not perform well in the long run. This would give an unfair advantage to the new variation.
In general, you should always watch out for factors that would affect each variant in a different way, even after randomization takes place. Remember – as Andrew pointed out before, the most dangerous word for marketers is “optimized.” The job is never really finished, and A/B testing can help us continue the process of optimizing.