The Dirty Truth About Clean Product Data
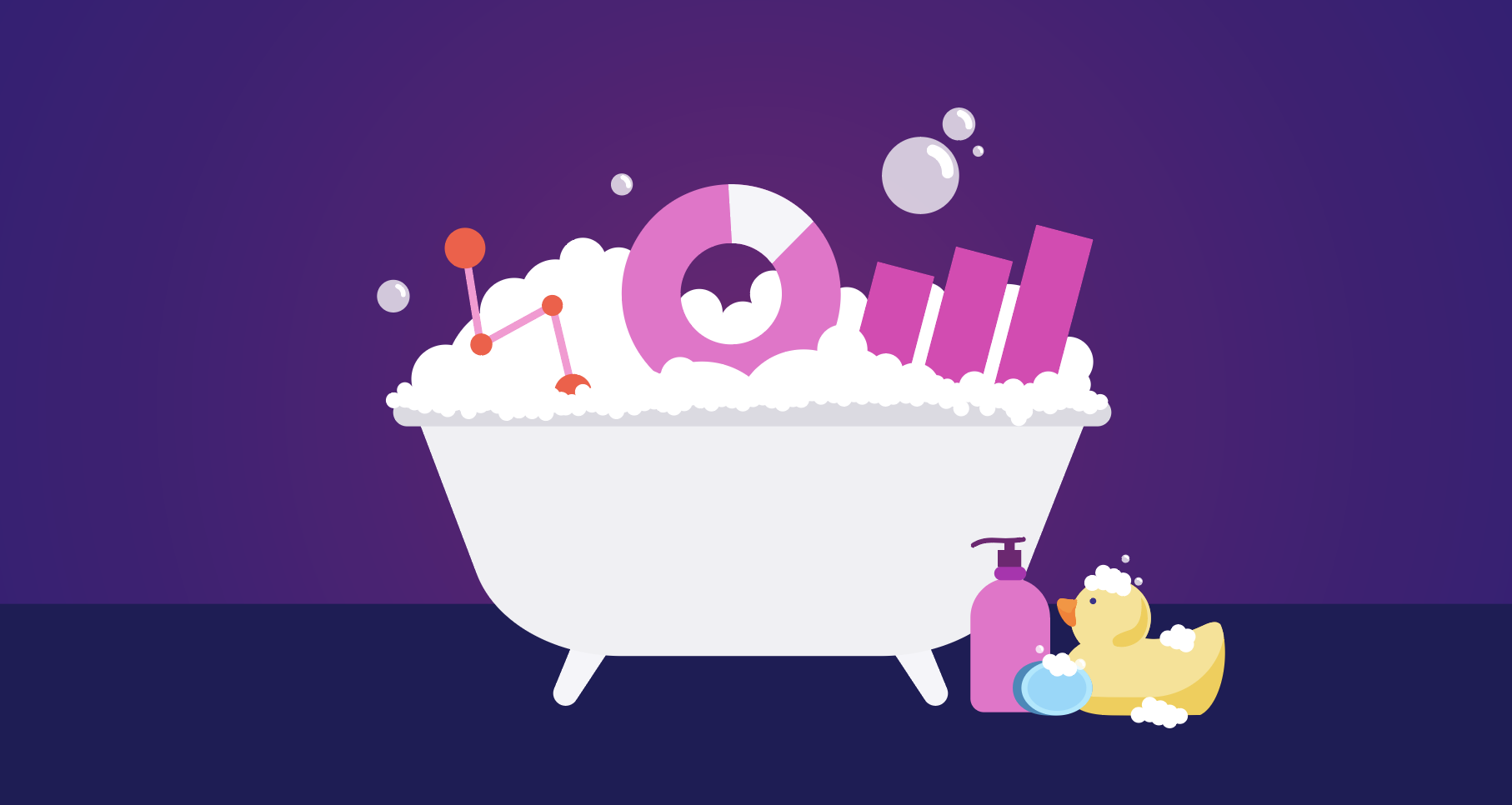
When you encounter a bad product experience, you know it. You could be looking for shoes, but there’s no preview image. Or you want to buy a new table for your dining room, but the product dimensions aren’t listed. All of this stems from bad product data. From a customer perspective, bad product data means a bad experience with your brand. From a business perspective, bad product data leads to a decrease in customer retention and lost revenue.
Keeping your product data clean and compliant is no easy feat. Often the process of cleaning data is expensive and time-consuming. But, having accurate product data is exceptionally important for consumers. It gives your customers all the information they want about a product and increases their confidence in doing business with you.
Because of the importance of high-quality data, skipping the cleaning process outright is not an option. So, how then can we limit the expensive impact of data cleaning? The best way is to make sure it gets done properly the first time. If you follow the steps in this article, you should be able to do just that.
What Makes Data Dirty?
There are a few different criteria that data analysts use to determine the quality of data they’re looking at, which we’ll examine now.
Data Validity
The first criterion is validity. Data validity acts as a gut-check to see if the data makes sense. The question you’ll be asking when checking for data validity is, “Does this data follow the rules?”
For example, when talking about shoe size, we expect all data to be a numeric value that is either a whole or half number. The value should fall into a narrow range between about 3 and 16 (for US Men’s sizes). These rules help to filter out bad data, but they will not tell you if your data is correct.
Data Accuracy
Data accuracy defines whether the data is correct. The problem with determining data accuracy is that it requires a secondary set of data with the “true” value for the data. There will be many cases where such a database is unavailable (or maybe that’s what you're trying to generate with this data cleansing exercise). In that case, you won’t be able to know for certain that the data you’re using is accurate.
Data Completeness
The next criterion of dirty data is completeness. Completeness will answer the question of “What data is missing?” In most cases, every single attribute will not need to be filled out for every single product. However, there will likely be a set of attributes that every single product should have: SKU, shoe size, color, brand, material, etc.
When any of these fields are empty, it’s easy enough to decide that the field should be populated with the correct value. However, if your shoe store sells track and field shoes, it may have an attribute of “track and field event” to help categorize the shoes. This attribute doesn’t really make sense on something like a sandal. Should the sandal have an empty or null value for the track & field event attribute? Should it be assigned a value of “N/A”?
There are a few different approaches for filling missing data, but the important thing is to ensure that the same approach is used across the entire dataset.
Data Consistency
Consistency of data is the next criterion for determining data cleanliness. Consistency means that data in one section of your catalog matches data in another section. If the shoe with SKU #12345 is listed as green in one section of your database but is listed as blue in another, it will be impossible to determine which of the values is correct.
Should a new value of blue/green or turquoise be added? Is one of the values distinctly wrong and needs to be replaced with the other? Just like with completeness, these questions are not trivial and should be made by someone that is close to the data. The important thing is to make sure changes are purposeful and uniform across the dataset.
Uniformity of the Data
The last thing to look at is the uniformity of the data. Uniform data uses the same format and units of measure across your dataset. If half of your shoes have sizes in US units, but the other half have sizes in European units, your customers are not going to be able to find what they are looking for and you will miss potential sales.
If someone who lives in the US saw shoes with a size of 45, they wouldn’t know whether the shoes would fit. Sure, you could look up a conversion, but why put in the effort when you can find a different place to purchase the same shoe?
Another major place where this appears is with dates. Is 12/10/2019 supposed to be Dec. 10th or Oct. 12th? If you were hoping to do a December holiday sale on a line of snowshoes, but the incorrect date format was used, your company could lose out on a lot of revenue.
Do You Have a Problem?
Where are you currently storing data? Many businesses store their data in spreadsheets, whether hosted through their eCommerce platform or in a program like Excel. This is all fine and well, but as your product data grows, managing from a spreadsheet can be harder to maintain.
There are a few ways you can run reports to see how accurate your data is currently, from running spot checks, calculating the percent completeness, and collecting feedback and user testing. Again, these are all good methods, but you’ll likely have challenges scaling these processes as your data continues to expand.
However you choose to do it, the point is that good product data can be taken for granted. It needs to be measured and corrected regularly.
Managing Going Forward
Determining if you need a data management solution will be different for every business, but typically the biggest factor to help you decide is how many product SKUs you have and how frequently you’re adding or removing products from your site. If the number of SKUs or frequency of updates is high for you, it’s time to consider a data management solution.
Fortunately, there are tools that exist to help you tackle all these product data problems. Product Information Management (PIM) systems allow you to set up attributes with designated types, which forces you to enter valid data. PIMs store your data in an easily accessible and easily readable format that acts as your source of truth for data accuracy.
Many PIMs have the ability to mark attributes as required, so you can automatically keep track of product data completeness. You’ll be able to define specific options for attributes like color. This will prevent inconsistencies from sneaking into your data through typos or other errors.
Finally, PIMs allow you to set units of measure, date formats, and other important components of uniform data. They will even let you set different units and formats for different locations.
There are several PIM platforms available depending on your data management needs. Akeneo, EnterWorks, Riversand, and Salsify are just a few of the major players in the PIM space. I recommend checking them out, not only for the sake of your data, but for the sake of your business as a whole.